Summarizing ecology from simple to complex organisms
Given the complexity of animal life forms and processes, it is impossible to reproduce their entire morphological space and how it is connected to their ecological success. With this project we propose an innovative solution employing deep learning, a method particularly adapt at complex task solving, to derive the ecological suitability of organisms. We base our model on a comprehensive dataset encompassing discrete and continuous morphological, ecological and life-history traits from different data-sources, species georeferenced occurrences (presences and absences) for a broad range of extant (and partly extinct) animal species of the phylum Chordata and the related climate conditions. Using our neural network architecture, we aim to capture the relationship between a species' position in the phenotypic space and their tolerance to abiotic factors (analogously to SDMs) but also additionally to model organism interactions such as prey-predator dynamics and competition. Ultimately, our goal is to identify which organisms are likely to inhabit a given cell based on this complex abiotic and biotic suitability function. These abiotic and trophic dependencies will inform the ecological function within the Gen3sis simulation model and will help in the exploration of the ecological and evolutionary factors that have shaped the vast diversity of organisms that we observe today.
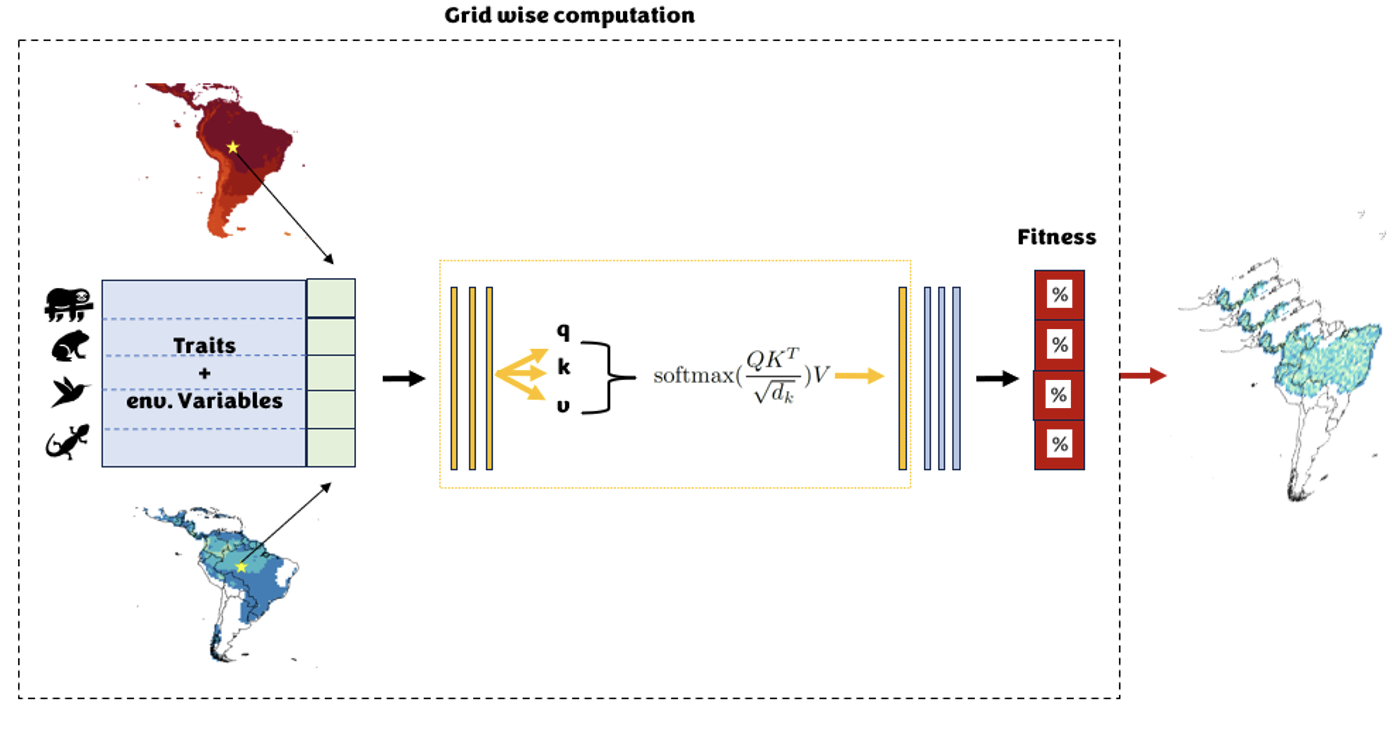
Contact information
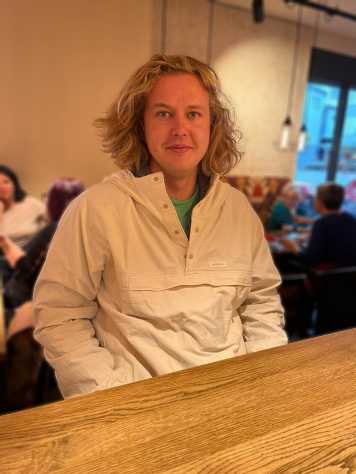
Thomas Toschini