DNai
Processing of environmental DNA using artificial intelligence for ecosystem monitoring
The current biodiversity crisis demands novel approaches to monitor how human activities influence the biosphere. The rapid development of 'omics' tools, in particular the metabarcoding of environmental DNA (eDNA), have opened a new area of comprehensive biodiversity data generation across many regions of the world. Yet, the development of efficient data processing pipelines has not matched the exponential increase of 'omics' data, which limits the application of eDNA for ecological monitoring. Processing eDNA requires multiple bioinformatic steps, with each step relying on poorly automatized disparate software, and many paths to choose with output results sensitive to subjective decisions. Moving toward large-scale biodiversity monitoring requires a fast, objective, and automated processing pipeline that will transform eDNA data into meaningful information about ecosystems including (i) standardized taxonomic lists for each sampled location that guide species management, (ii) standardized classification of samples from their DNA composition which can guide ecosystem management. In this project, we propose to harness a combination of machine learning approaches that transforms eDNA metabarcoding data into informative ecological indicators that improves ecosystem monitoring and decision making. As interpretation from eDNA signal is traditionally generated from the association of the DNA reads to taxonomic labels, we will first develop a machine learning method to associate sequences with a reference database and identify the taxonomic composition of eDNA in samples. Second, we will use unsupervised machine learning in order to ordinate the high dimensional ensemble of DNA reads from eDNA into reduced latent dimensions, which reflect ecosystem properties. We will finally evaluate whether supervised learning can predict ecosystem properties of interest directly from DNA sequences composition in eDNA samples.
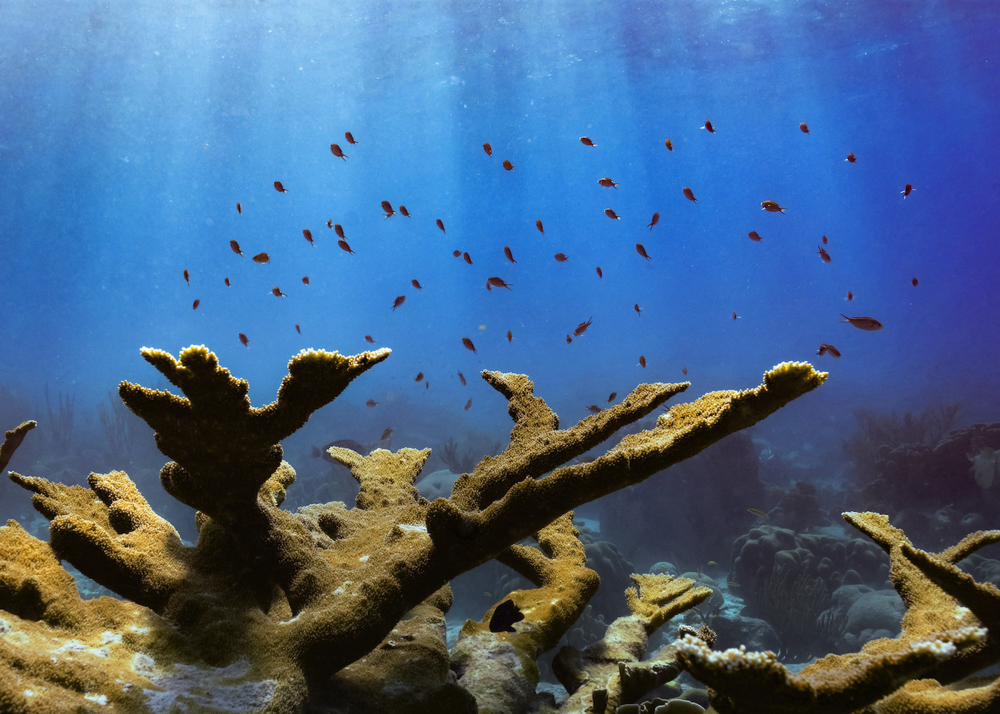
Those developments will be done from two global marine datasets gathering eDNA samples collected in multiple sites across all oceans, from the Antarctic to tropical seas, which are available for analyses, targeting (i) marine fishes and (ii) marine prokaryotes and eukaryotes. Key milestones of the project include: (a) an operative pipeline using machine learning-based sequence recognition to taxonomic labels; (b) learning a dimensional reduction of complex eDNA reads composition into a set of indicators for ecosystem quality and functioning; (c) training models to relate eDNA composition to complex ecosystem properties, related to health and productivity.