Eco-evolutionary processes in ecological and economic systems
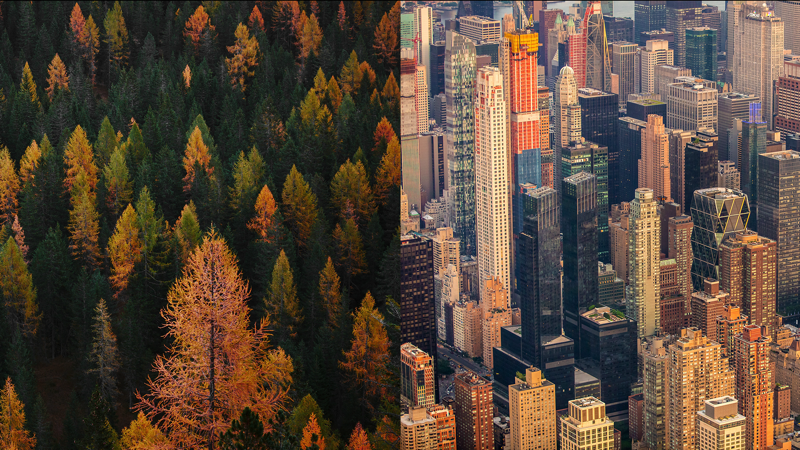
Ecological and economic systems are complex adaptive systems (CAS): they are systems that are composed of many entities with heterogeneous characteristics, which interact and experience selection processes. Those processes act at the entity level, but are key in determining the macroscopic behaviour at the system level, a feature that make those systems unique. For instance, the diversity of species within an ecosystem results from a hierarchy of processes acting at different scales of time and space, comprising the variations experienced by single organisms, their interactions, and selection pressure acting upon populations. Analogously, economic growth at the level of a country is greatly affected by its ensemble of economic actors, their interactions, and the selection pressure they experience. Despite the complexity of the processes driving their dynamics, regularities at the macroscopic level emerge in ecological and economic systems. This is the case of large-scale spatial patterns of biodiversity and differences in economic growth across countries, calling for a mechanistic understanding of the essential mechanisms that generate them.
Recently, a considerable interest has grown up around the interplay between ecological processes, the processes that regulate interactions between organisms, and evolutionary processes, the change of the characteristics of biological populations over time, to explain current biodiversity patterns. Analogous economic processes have been proposed to explain differences in economic growth across nations. Nonetheless, a quantitative investigation of their importance is missing. Determining how those patterns can emerge from eco-evolutionary processes is required to improve our current understanding. This project delivers new quantitative insights following a unique approach that combines dynamical eco-evolutionary models and empirical data.
Simulations of eco-evolutionary models and their integration with empirical data pose several methodological challenges that we adress in the first part of this project. Entities in CAS have distinct quantitative attributes that determine their fitness in a given environment. Accounting for the variety of these characteristics leads to models with a high dimensionality, associated to a large if not prohibitive computational cost preventing its simulation. In particular, partial differential equation (PDE) models, which can encode eco-evolutionary processes acting upon entities defined by many characteristics, are cursed by their dimensionality. To this aim, we develop machine learning algorithms that break down the curse of dimensionality. Such algorithms rely on neural networks to approximate the solution to PDE models. An other difficulty consists in confronting eco-evolutionary models outputs to data, since those models cannot be manipulated with standard statistical techniques. We apply methods commonly employed in the training of neural networks, together with model selection techniques, to infer fundamental mechanisms that might have generated the patterns under investigation. Altogether, the methods permit efficient model simulations and their integration with empirical data, allowing to deliver quantitative answers to the motivated research questions.
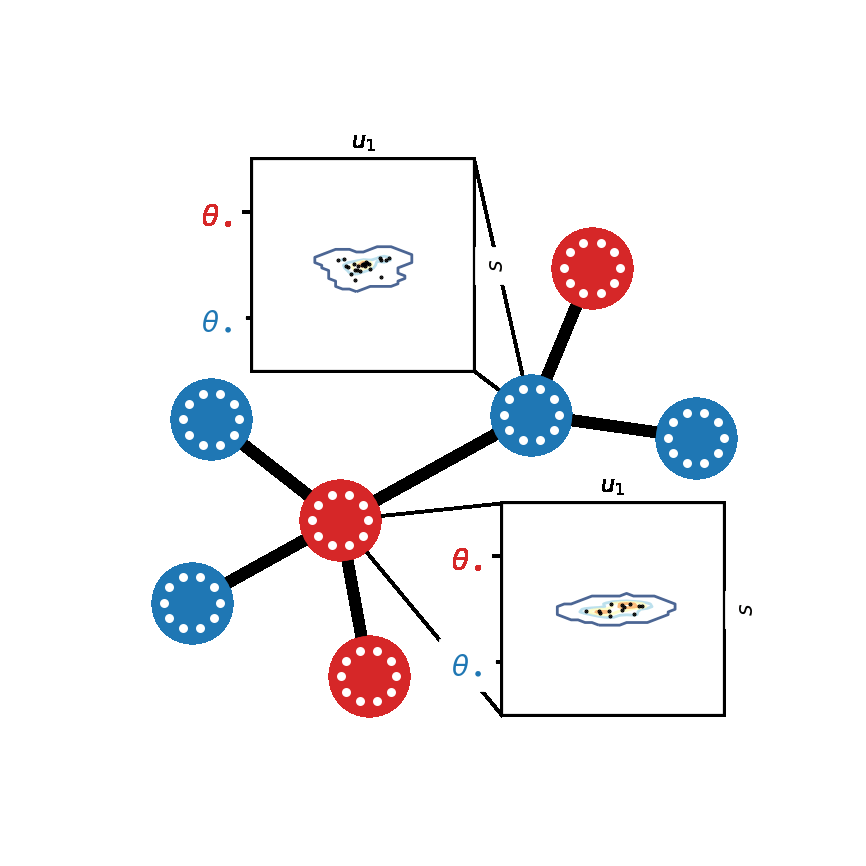
In the second part, we make use of the above techniques to study eco-evolutionary models and to test them against data, to explore hypotheses on the fundamental mechanisms that drive patterns of biodiversity and economic growth. From one hand, we explore how eco-evolutionary processes, in combination with complex landscape topologies, can explain patterns of species diversity. To this aim, we develop and analyse an eco-evolutionary model on spatial graphs, to understand how the combination of eco-evolutionary processes and spatial structure might have shaped biodiversity patterns that are found in complex landscapes such as mountain regions.
On the other hand, we investigate how eco-evolutionary processes can provide new insights in the understanding of economic dynamics. We proceed by developing a simple eco-evolutionary model which explanatory power we test against long time series that proxy the dynamics of the size of economic sectors.
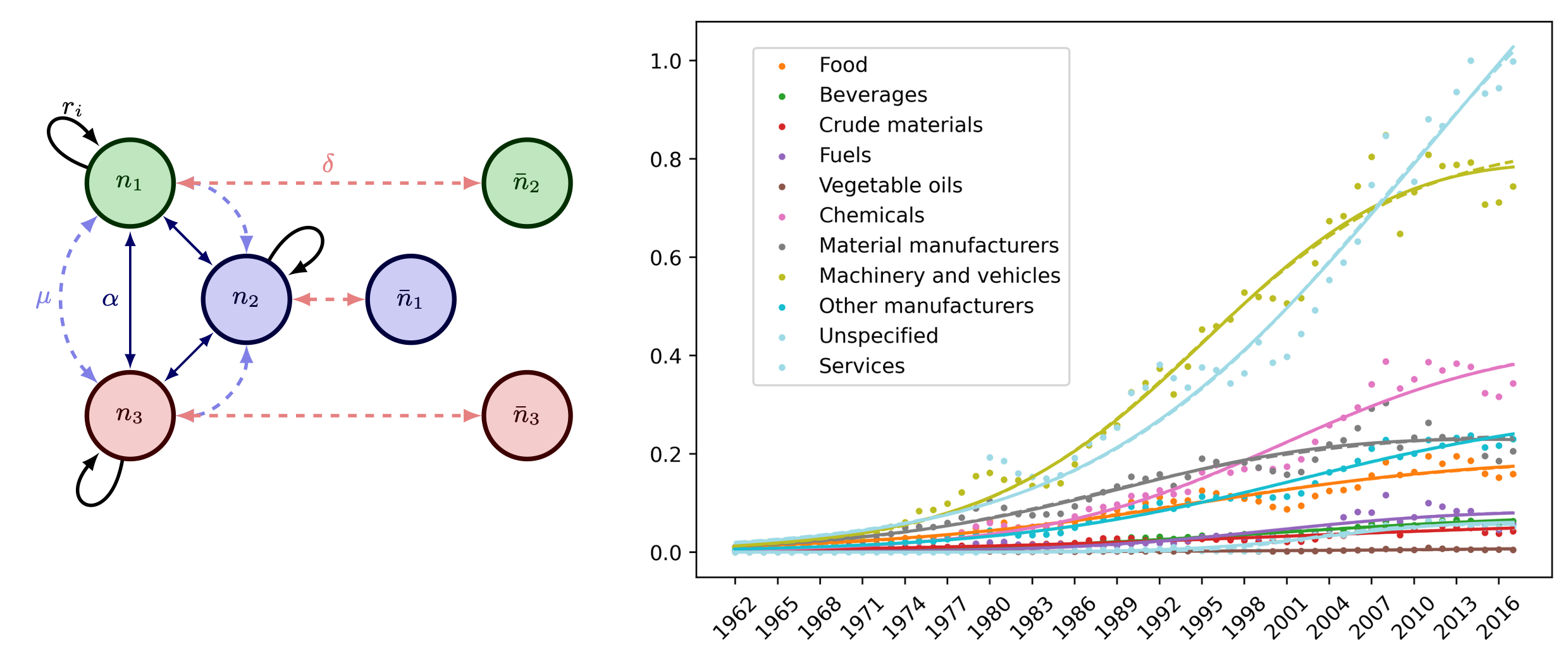
Overall, this project delivers quantitative insights on how the interplay between ecological and evolutionary processes is shaping the features of the world that surrounds us.
Contact information